Why HBM4e is Key to Unlocking the Next Era of AI Innovation
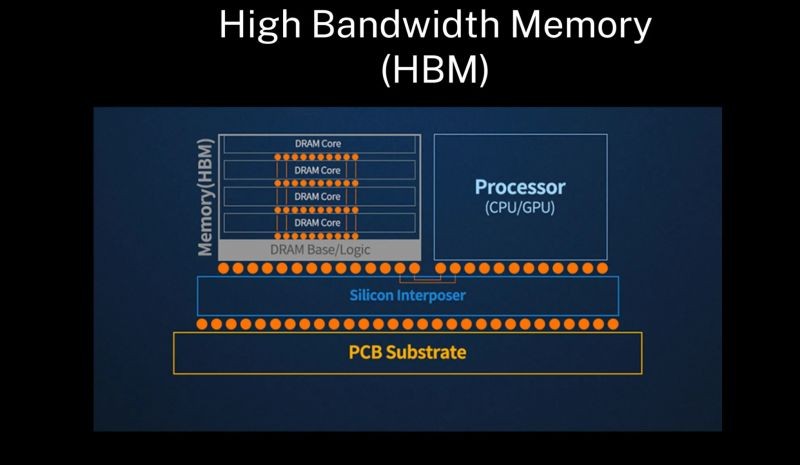
Faster_Data_Processing:
AI models, especially deep learning models, require vast amounts of data to be processed quickly. HBM can dramatically reduce data transfer bottlenecks, enabling faster training and inference times for models.
Higher_Bandwidth:
Modern AI applications, such as those in natural language processing (NLP), computer vision, and autonomous systems, demand very high bandwidth to keep pace with the massive data flowing between processors and memory.
Energy_Efficiency:
AI models, particularly large ones, require a tremendous amount of power to compute. HBM reduces energy consumption compared to traditional memory systems while offering better performance.
Impact of HBM4e in AI Forecast:
If HBM4e (or its equivalent) were to emerge as the next-generation memory technology, the forecast for AI would likely include the following:
Increased Training Speed for Larger Models:
AI models, particularly deep neural networks, continue to grow in complexity and size.
Improved_Real_Time AI Applications:
Applications like self-driving cars, real-time language translation, and augmented reality require fast, high-bandwidth memory solutions. With the improved performance of HBM4e, real-time AI could become even more efficient and responsive.
More_Scalable AI Solutions:
Memory improvements help make AI applications more scalable. As the memory system becomes more capable of handling larger datasets and more complex models, AI systems can scale efficiently to tackle real-world problems at an industrial level.
Broader_Adoption of AI in New Fields:
With faster and more efficient memory, industries like healthcare, finance, robotics, and manufacturing will be able to leverage more sophisticated AI models, leading to new breakthroughs in predictive analytics, robotic automation, personalized medicine, and more.
Potential_Future_Developments with HBM4e:
Beyond HBM2: HBM2 and HBM3 are already in use in AI and high-performance computing. The next generation (like HBM4e) would likely offer even faster speeds, lower latencies, and better power efficiency.
AI_Specific_Optimizations:
HBM4e could be designed to handle the specific demands of AI, such as parallel processing and massive data throughput, more effectively than previous versions.
Integration with AI Accelerators:
AI-specific hardware like ASICs and FPGAs could integrate HBM4e directly into their design, leading to even more powerful specialized AI systems.
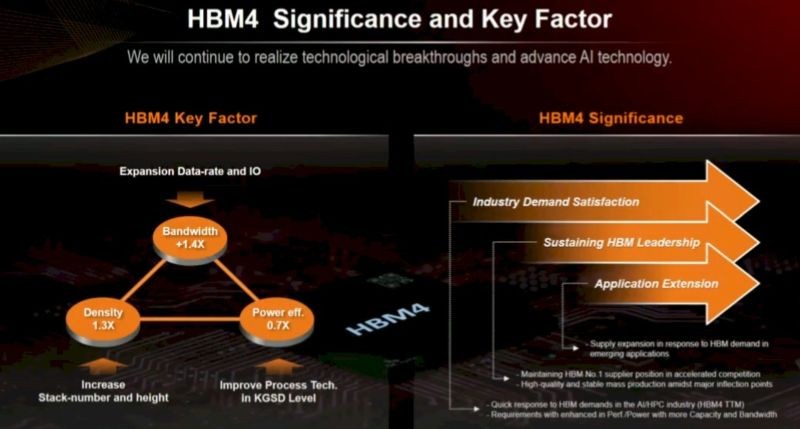
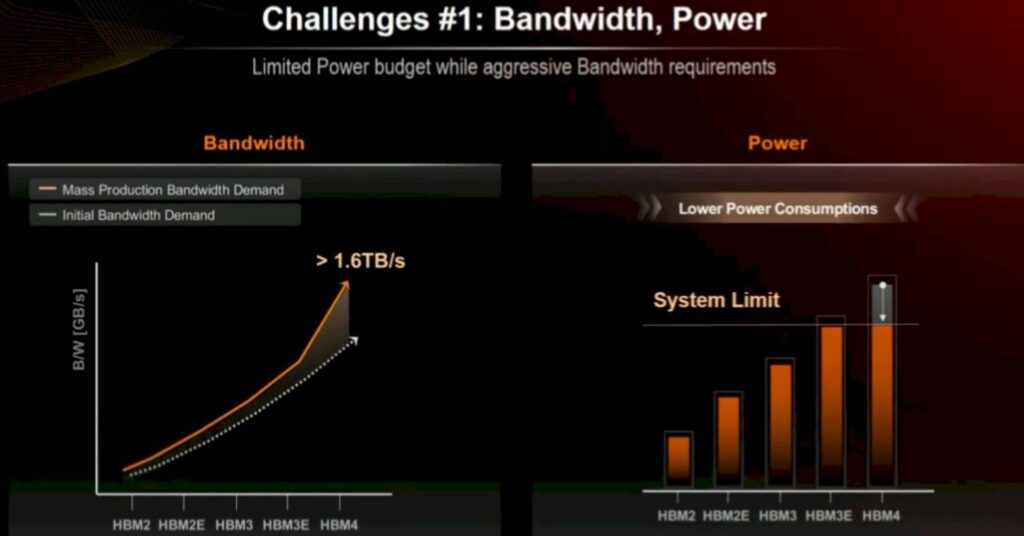
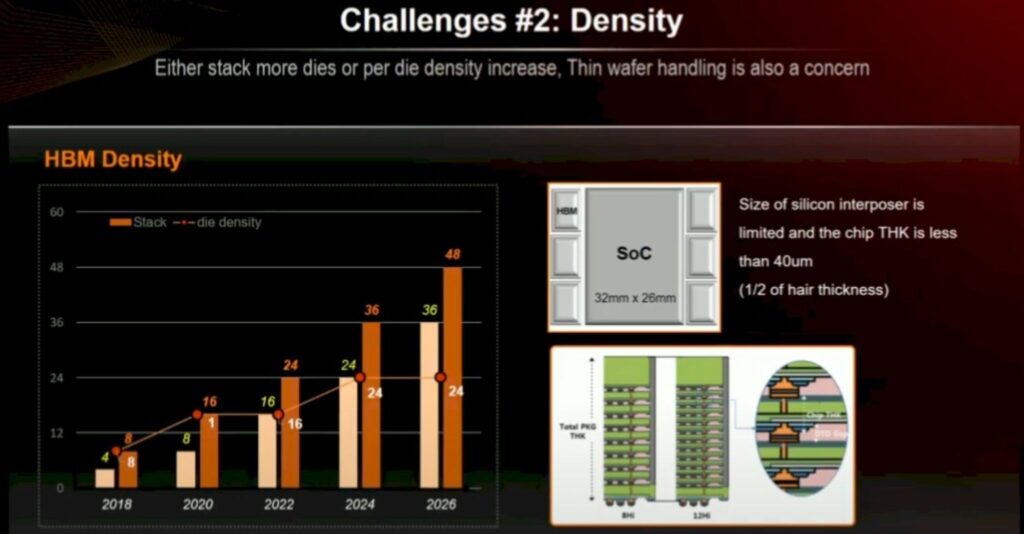
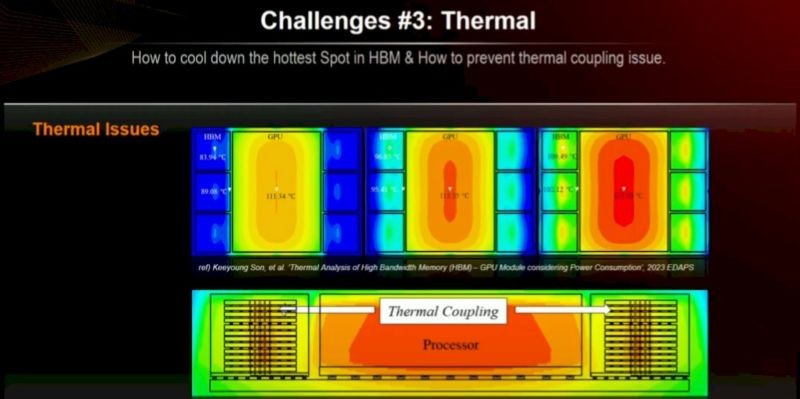
Conclusion:
While HBM4e is not yet a standard, the ongoing development of high-bandwidth memory technologies, including potential advancements like HBM4e, will be crucial for the future of AI. Faster memory systems are essential to support the ever-increasing demands of AI models, improving speed, scalability, and energy efficiency.
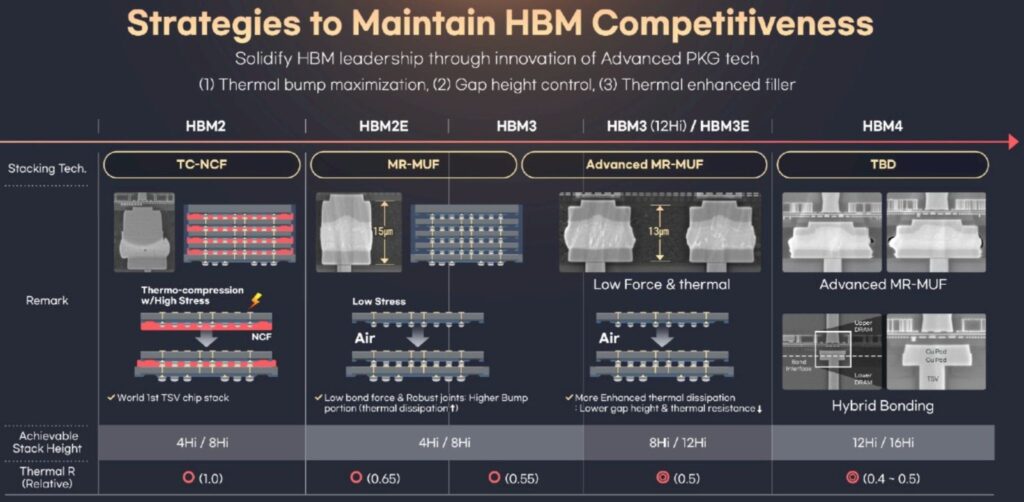